Get my own profile
Public access
View all11 articles
0 articles
available
not available
Based on funding mandates
Co-authors
Ziwei JiGoogle ResearchVerified email at google.com
Daniel HsuColumbia UniversityVerified email at cs.columbia.edu
Sham M KakadeHarvard UniversityVerified email at seas.harvard.edu
Anima AnandkumarCalifornia Institute of Technology and NVIDIAVerified email at caltech.edu
Rong GeDuke UniversityVerified email at cs.duke.edu
Peter BartlettProfessor, EECS and Statistics, UC BerkeleyVerified email at cs.berkeley.edu
Dylan J. FosterPrincipal Researcher, Microsoft ResearchVerified email at microsoft.com
Maxim RaginskyProfessor of Electrical and Computer Engineering, University of Illinois, Urbana-ChampaignVerified email at illinois.edu
Alexander RakhlinProfessor, MITVerified email at mit.edu
Bolton BaileyPh.D. Candidate, University of Illinois, Urbana-ChampaignVerified email at illinois.edu
Robert SchapireMicrosoft ResearchVerified email at microsoft.com
Miroslav DudikMicrosoft ResearchVerified email at microsoft.com
Andrea VattaniChief Scientist at Spiketrap, Computer Science PhD from UC San DiegoVerified email at cs.ucsd.edu
Clayton SanfordColumbia UniversityVerified email at columbia.edu
Nathan SrebroProfessor, TTIC and University of ChicagoVerified email at ttic.edu
Jacob AbernethyAssistant Professor, University of MichiganVerified email at umich.edu
Sébastien LahaieGoogle ResearchVerified email at google.com
Ruta MehtaDept. of Computer Science, University of Illinois at Urbana-ChampaignVerified email at cs.illinois.edu
John LangfordMicrosoft Research New YorkVerified email at hunch.net
Alekh AgarwalGoogleVerified email at google.com
Follow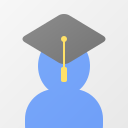
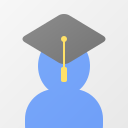
Matus Telgarsky
Courant Institute of Mathematical Sciences, New York University
Verified email at nyu.edu - Homepage